AWS에서의 Apache MXNet은 빠르고 확장 가능한 훈련 및 추론 프레임워크로서 기계 학습을 위해 사용이 쉽고 간단한 API가 제공됩니다.
MXNet에는 Gluon 인터페이스가 포함되어 있으므로 모든 기술 수준의 개발자가 클라우드, 엣지 디바이스 및 모바일 앱에서 딥 러닝을 시작할 수 있습니다. Gluon 코드 몇 줄로 객체 감지, 음성 인식, 추천 및 개인화를 위한 선형 회귀, 컨벌루션 네트워크 및 반복 LSTM을 구축할 수 있습니다.
대규모로 기계 학습 모델을 구축, 교육 및 배포할 수 있는 플랫폼인 Amazon SageMaker를 사용하면 AWS에서 완전 관리형 MxNet 환경을 시작할 수 있습니다. 또는 AWS Deep Learning AMI를 사용하여 MxNet의 사용자 정의 환경 및 워크플로를 비롯해 TensorFlow, PyTorch, Chainer, Keras, Caffe, Caffe2 및 Microsoft Cognitive Toolkit 같은 기타 프레임워크를 구축할 수 있습니다.
MXNet를 사용한 딥 러닝의 이점
Gluon의 사용 편의성
뛰어난 성능
IoT 및 엣지용
유연성 및 선택권
고객 수요
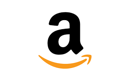
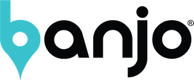
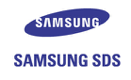

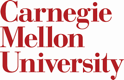
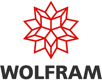
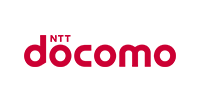
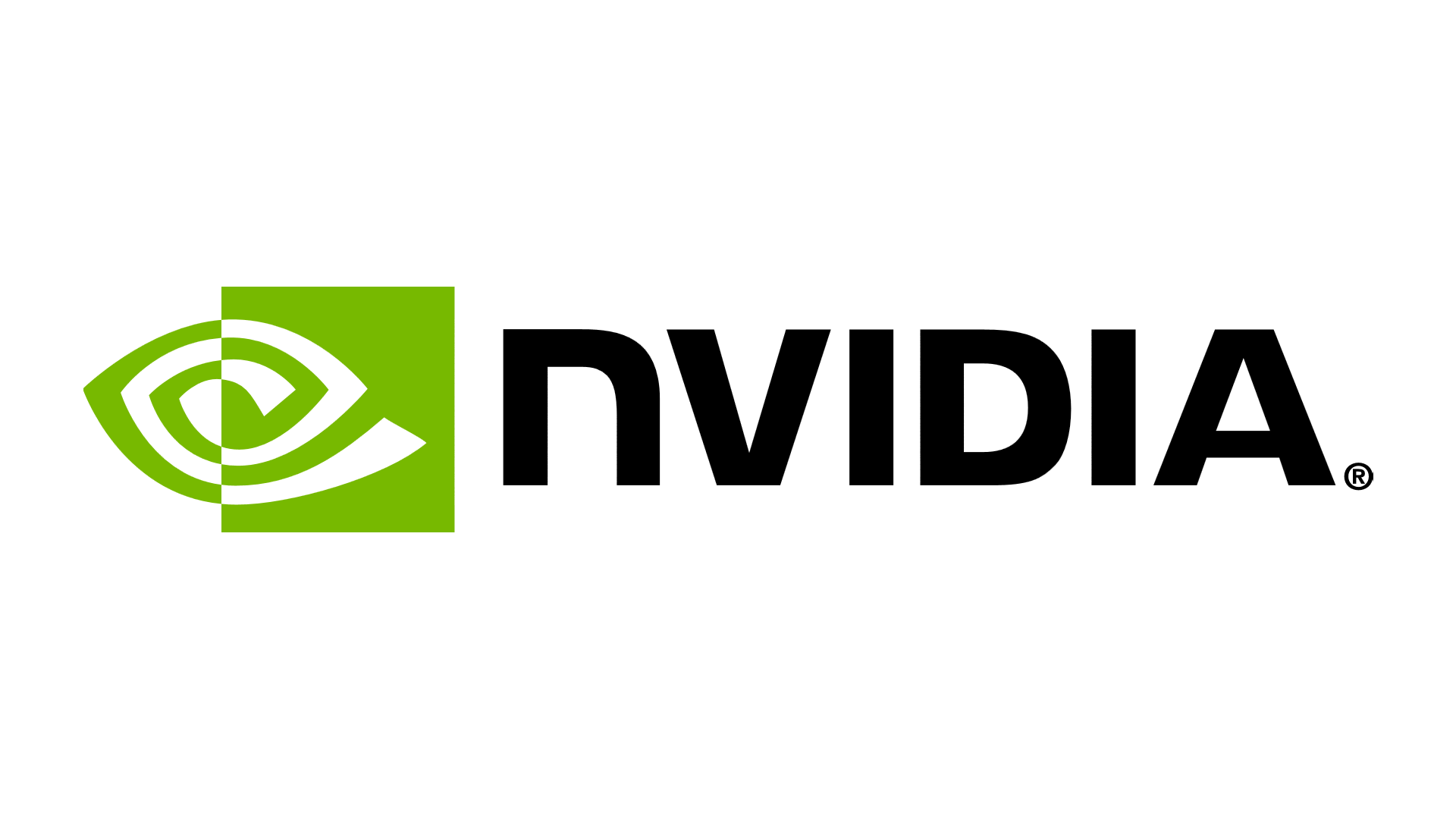
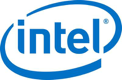
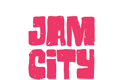
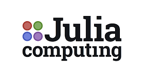

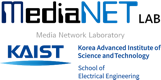

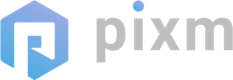
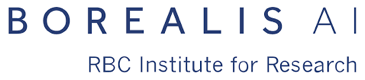

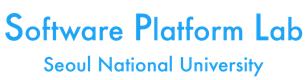
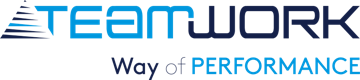
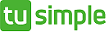

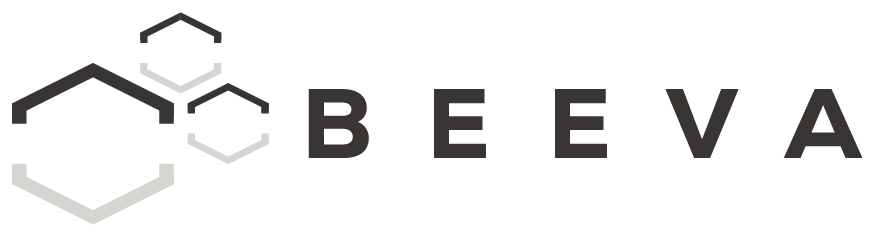
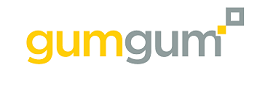
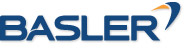
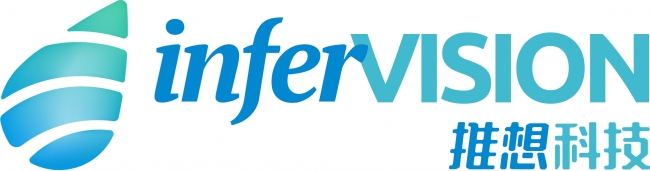
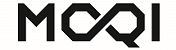


사례 연구
Amazon, NVIDIA, Intel, Samsung 및 Microsoft의 개발자를 비롯하여 500명 이상이 MXNet 프로젝트에 참여했습니다. 다른 고객들이 딥 러닝 프로젝트에 MXNet을 어떻게 활용하고 있는지 알아보십시오. AWS Machine Learning 블로그와 MXNet 블로그에서 더 많은 사례 연구를 참조할 수 있습니다.
기계 학습을 위한 Amazon SageMaker
Amazon SageMaker는 개발자 및 데이터 과학자가 모든 규모의 기계 학습 모델을 쉽고 빠르게 구축, 교육 및 배포할 수 있게 지원하는 완전관리형 서비스입니다. Amazon SageMaker는 기계 학습을 사용하려는 개발자가 일반적으로 빠른 속도를 내지 못하게 하는 모든 장벽을 제거합니다.