Customer Stories / Life Sciences / Americas
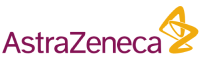
AstraZeneca Accelerates Time to Insights Using Amazon SageMaker
AstraZeneca used AWS to build a commercial analytics solution in 2.5 months that uses Amazon SageMaker to automate machine learning model development and deployment and accelerate time to insights.
5 minutes
Creates an ML development environment for data scientists in 5 minutes versus 1 month
2.5 months
Built a solution in 2.5 months compared to 6+ months previously
100 Data Scientists
Supports more than 100 data scientists
Reduces manual workload
for data scientists
Overview
Much of the health and life sciences industry is faced with an ever-increasing amount of commercial data and struggles to efficiently analyze it. AstraZeneca, the science-led biopharmaceutical company, is no different. In managing the vast and ever-increasing amount of data, the company realized it was missing an opportunity to gain valuable business insights about its treatments. It needed an efficient development process to create and deploy machine learning (ML) models into production, rapidly analyze the data at scale, and generate business insights that would help it improve research and development and accelerate commercialization of new therapeutics, ultimately speeding up delivery of life-changing medicines to patients.
AstraZeneca worked with Amazon Web Services (AWS) to build a solution using Amazon SageMaker, which helps data scientists and developers prepare, build, train, and deploy ML models quickly. Now, AstraZeneca not only analyzes the commercial data at scale to gain insights but also accelerates insights by automating much of the previously manual processes, saving time and effort for its data scientists.
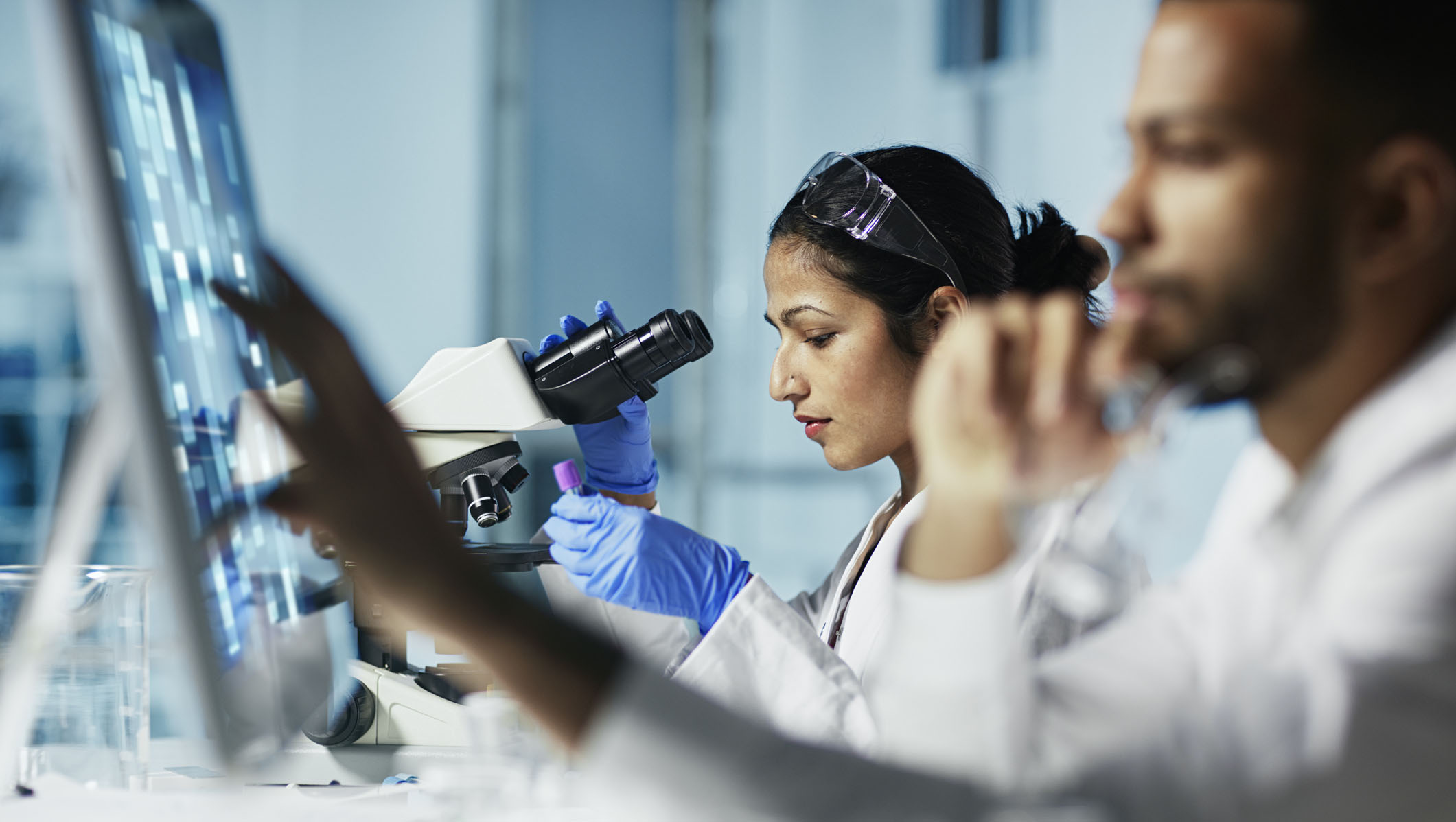
Opportunity | Using ML on AWS for Large-Scale Data Analytics
As a global, science-led company, AstraZeneca is focused on the discovery, development, and commercialization of life-changing medicines in oncology, rare diseases, and biopharmaceuticals for the cardiovascular system and renal and metabolic function, as well as respiratory health and immunology, supporting millions of patients across 145 countries and 70 markets. AstraZeneca analyzes anonymized patient data to gain insights including learning patient sentiments on specific treatments and feeding progression models on therapeutic areas such as chronic kidney disease, heart failure readmission, and cancer classification. AstraZeneca delivers those insights to commercial analysts, who in turn use them to improve business processes and drive awareness and uptake with healthcare systems. “We focus on targeting alignment and marketing, helping our commercial teams deliver the right information to healthcare providers to address patient needs,” says Cherry Cabading, global senior enterprise architect at AstraZeneca.
Delivering those insights requires the analysis of large datasets. And like many large corporations, AstraZeneca’s commercial team didn’t have an agile ML environment in which to process the data at scale. Its prior ML solution required over a month of intensive effort to establish an environment for data scientists that would give them access to the necessary data. This was inefficient in both time and cost. “We previously used a technology stack that lacked an automated way of producing the environment for a team of data scientists,” explains Cabading. “It was hard to even glue it together because there was no cohesion among the various ML tools available in the market.”
In 2020, the commercial team was looking for a solution that would provide extensibility, flexibility, and scalability. “The data scientists required a solution for building, training, and deploying ML models then industrializing those models to be integrated with our commercial system,” says Cabading. By using Amazon SageMaker, AstraZeneca was able to implement a standard, unified solution for ML development, avoiding months of writing custom integration code while also reducing cost.

Rather than creating many manual processes, we can automate most of the machine learning development process simply within Amazon SageMaker Studio.”
Cherry Cabading
Global Senior Enterprise Architect, AstraZeneca
Solution | Increasing Speed, Efficiency, and Simplicity on AWS
In 2020, Cabading and the team began architecting and designing the solution using Amazon SageMaker. Just as the team was preparing to implement ML operations, AWS released Amazon SageMaker Studio, a fully integrated development environment for ML that brings everything needed to prepare data and build, train, and deploy models under one unified web-based visual interface. AstraZeneca began using Amazon SageMaker Studio in July 2021. “It includes the deployment, the ML operations, the model registry, the SageMaker Feature Store, and the ability to deploy the models to different stages or environments,” says Cabading. “Rather than creating many manual processes, we can automate most of the ML development process simply within Amazon SageMaker Studio.”
AstraZeneca’s ML solution, called Advanced Insights Generator (AIG), helps analysts carry out commercial modeling. In the US commercial sector, AIG is used with science teams comprising over 100 data scientists. Data scientists request an environment and the data they want to access, such as biopharmaceutical or oncology data. To manage those requests, Cabading’s team uses AWS Service Catalog, which provides a single location where organizations can centrally manage catalogs of information technology services. “We enter the name of the data science team and select a checkbox for which data they want to access,” says Cabading. Then, a new environment automatically launches. The entire process takes 5 minutes, compared to more than a month previously, so data scientists can focus on more valuable work and deliver insights rapidly.
Using AIG, the commercial team can generate insights in less than 2.5 months compared to over 6 months previously, an approximately 150 percent improvement in time to insights. “Because the solution has infrastructure as code, it’s simple to repeat. We don’t need to reinvent the wheel for those projects,” says Cabading. “We can share this solution across different internal and external partners where it makes the most sense.”
Outcome | Extending the Amazon SageMaker Solution Globally
Currently, AIG is used within the US commercial market and by Global Medical. AstraZeneca expects to share the AIG capabilities more broadly, both internally and with key external partners.
By using Amazon SageMaker and other AWS services, AstraZeneca was able to rapidly deploy a solution to analyze large amounts of data, accelerating insights while reducing the manual workload of its data scientists—crucial to AstraZeneca’s mission of discovering and developing life-changing medicines for people around the world.
About AstraZeneca
AstraZeneca discovers, develops, and commercializes prescription medicines in oncology and biopharmaceuticals, including in cardiovascular, respiratory, and immunology fields. It serves millions of patients across 145 countries and 70 markets.
AWS Services Used
Amazon SageMaker
Amazon SageMaker helps data scientists and developers to prepare, build, train, and deploy high-quality machine learning (ML) models quickly by bringing together a broad set of capabilities purpose-built for ML.
Amazon SageMaker Studio
Amazon SageMaker Studio provides a single, web-based visual interface where you can perform all ML development steps, improving data science team productivity by up to 10x.
AWS Service Catalog
AWS Service Catalog allows organizations to create and manage catalogs of IT services that are approved for use on AWS. These IT services can include everything from virtual machine images, servers, software, and databases to complete multi-tier application architectures.
More Life Sciences Customer Stories
Get Started
Organizations of all sizes across all industries are transforming their businesses and delivering on their missions every day using AWS. Contact our experts and start your own AWS journey today.